Operational Efficiency
The Operational Efficiency Challenge
Meeting the challenges of ensuring the efficient and economical running of an investment management business continue to be a primary focus among our clients. Increased demands for high quality and timely information from stakeholders including Regulators, Clients, Senior Management and Front Office demand systems and processes are implemented to create a symbiotic relationship between them and human resources used to oversee rather than produce output; ensuring quality through utilising their knowledge and experience in combination with process and technology.
Each client’s operational landscape is unique and needs to be understood in order to engage in the task of creating a framework in which throughput can be managed and made scalable in an economical way while maintaining control and transparency.
The challenge for investment managers is to balance the the need for scalability against the organic way many operational landscapes have evolved. ISC has the experience and knowledge to face this challenge with our clients.
Resolving The Challenge
ISC has a deep history of delivering successful projects providing operational improvement and transformation. This spans wholesale systems selection and implementation to in-depth analysis of operational areas and re-design of robust, scalable processes to ensure operational challenges are overcome.
Our experience as programme and project managers, business analysts and SMEs ensures understanding of the project management process.
ISC understands the operational complexities underlying the processes supporting every step of the Investment Managers business from ensuring the smooth delivery of product, the lifecycle of transactions, through to the production and delivery of internal and external reporting. We engage with the client from a position of a holistic understanding of the business and use our experience to deliver pragmatic, timely and economical solutions.
Examples of our Completed Projects
An investment management client had a requirement to outsource internal functions as part of a firm-wide efficiency programme to provide cost savings to shareholders.
ISC's long established client required consultants to manage the transition of their Private Client book of business to another asset manager, including all assets, clients, personnel, data and records.
ISC's client required a rethink on how new funds were launched. The limitations of their funds' launch process was eroding the competitiveness of their funds range.

We listen to your needs
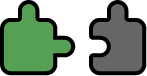
We understand your challenges
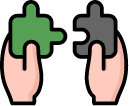
We provide solutions
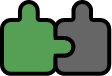
We help with implementation