The Benefits and Challenges of AI in KYC & CDD
"Machine learning models are only as good as the data they are trained on. Poor data quality leads to poor decisions." - Andrew Ng, AI Expert and Co-founder of Google Brain
AI’s Double-Edged Sword in Compliance
Artificial Intelligence is reshaping compliance in ways that were unimaginable a decade ago. Financial institutions are leveraging AI to enhance efficiency, reduce costs, and improve fraud detection in Know Your Customer (KYC) and Customer Due Diligence (CDD) processes. However, as AI-driven compliance becomes more prevalent, new challenges emerge, from ethical concerns to regulatory scrutiny.
AI’s role in compliance is both revolutionary and complex. While it brings undeniable advantages, institutions must navigate the challenges carefully to ensure that AI delivers on its promise without introducing new risks.
The Power of AI in Compliance
One of AI’s biggest advantages is its ability to process vast amounts of data in real time. Unlike traditional compliance systems that rely on rule-based logic and manual reviews, AI models continuously learn and improve, making them far more effective in identifying anomalies, detecting fraud, and assessing customer risk.
Automated document verification is one of AI’s most impactful applications. Optical Character Recognition (OCR) and facial recognition technologies streamline onboarding, reducing manual intervention and eliminating human errors. Customers can now verify their identities remotely, eliminating the delays and inefficiencies of paper-based processes.
Beyond identity verification, AI-driven transaction monitoring is transforming risk detection. Machine learning models can analyse millions of transactions per second, identifying unusual patterns that could indicate fraud, money laundering, or other financial crimes. Unlike static rule-based systems, AI adapts over time, reducing false positives and ensuring that compliance teams focus on genuine threats.
Another critical area where AI is making a difference is adverse media screening. Traditional methods required compliance officers to manually sift through news sources and databases, often leading to outdated or missed information. AI, powered by Natural Language Processing (NLP), scans global news reports, regulatory announcements, and even social media for potential red flags—providing institutions with real-time insights into emerging risks.
AI does not just stop at fraud detection; it also brings significant cost-saving benefits. Compliance processes that once required extensive human resources can now be automated, reducing operational expenses and allowing financial institutions to scale their compliance efforts without increasing headcount.
The Challenges of AI in Compliance
While AI is revolutionising compliance, its integration into KYC and CDD processes comes with significant hurdles. One of the most pressing concerns is the issue of false negatives in anomaly detection. AI models are trained on historical data, which allows them to identify known patterns of fraudulent activity. However, financial criminals are constantly evolving their tactics, developing new methods that may not fit previous fraud patterns. This means AI systems can sometimes overlook emerging threats, allowing illicit transactions to slip through undetected. The only way to mitigate this risk is through continuous model retraining and frequent updates, which adds to the operational complexity and costs of maintaining AI-driven compliance.
Beyond the challenge of keeping AI models up to date, financial institutions also face significant resource and investment barriers. Implementing AI-powered compliance tools requires a robust data infrastructure, advanced computing capabilities, and a team of highly skilled professionals, including data scientists and AI specialists. While large financial institutions may have the resources to build and manage these systems in-house, smaller firms often struggle with the steep upfront costs. Many turn to third-party AI providers to bridge the gap, but this introduces another layer of complexity—relying on external vendors brings concerns about security, vendor management, and regulatory accountability.
The effectiveness of AI in compliance also depends on the quality and completeness of data. AI models can only make accurate decisions when they are trained on high-quality, comprehensive datasets. However, many financial institutions face challenges with fragmented or inconsistent customer data, often stored in separate internal systems or data silos. If the data is incomplete or outdated, AI models may generate inaccurate risk assessments, leading to errors in identity verification or transaction monitoring. To fully unlock AI’s potential, organisations need to prioritise data integration strategies, ensuring that AI has access to a broad, well-structured dataset.
Adding to these technical and operational challenges is the issue of regulatory uncertainty. As AI becomes more deeply embedded in compliance processes, regulators are scrambling to establish clear guidelines on its use. Financial institutions must ensure that their AI systems meet regulatory expectations around transparency and explainability—two areas where AI, particularly machine learning, has often struggled. Regulators are increasingly demanding that financial institutions provide clear explanations of how AI-driven decisions are made, making the development of Explainable AI (XAI) a growing priority. Institutions that fail to meet these requirements could face increased scrutiny or even non-compliance penalties.
Finally, ethical concerns around bias in AI remain a major consideration. If AI models are trained on biased historical data, they can inadvertently reinforce existing disparities, disproportionately flagging individuals or businesses from certain demographics or geographic regions as high-risk. Addressing these concerns requires financial institutions to proactively audit their AI systems, implementing safeguards that ensure fairness and accountability. Transparency in AI decision-making is not just a regulatory requirement—it is also essential for maintaining public trust in financial institutions and their compliance practices.
Striking the Right Balance
The integration of AI into KYC and CDD processes is a balancing act. While AI offers unprecedented speed and efficiency, financial institutions must navigate the complex challenges of evolving fraud tactics, regulatory pressures, data dependencies, and ethical considerations. The institutions that succeed will be those that take a proactive approach—continuously refining their AI models, investing in high-quality data, and embracing ethical AI development. The future of compliance is not just about automation; it is about building AI systems that are transparent, fair, and adaptable to the ever-changing financial landscape.
AI is undeniably transforming KYC & CDD, offering speed, accuracy, and efficiency that manual processes simply cannot match. However, false negatives, excessive costs, data dependencies, and regulatory uncertainties present significant challenges that financial institutions must carefully manage.
The key to success lies in continuous monitoring, investment in high-quality data, regulatory adaptability, and ethical AI deployment. By addressing these challenges head-on, financial institutions can maximise AI’s potential while maintaining compliance and trust.
As AI continues to evolve, the future of KYC & CDD will be defined by institutions that balance innovation with responsibility.
In the next article, we will explore real-world applications of AI in KYC & CDD, highlighting how financial institutions are leveraging AI to enhance compliance, detect fraud, and improve customer experience.
What challenges has your organisation faced in implementing AI-driven compliance solutions? Share your insights in the comments.

We listen to your needs
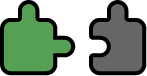
We understand your challenges
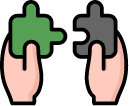
We provide solutions
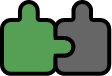
We help with implementation